The Client
Our client, a key market participant in the online food delivery market, realized the need to estimate Hong Kong consumers' interest in food delivery services. They used our restaurant data scraping services to collect data from OpenRice. Using OpenRice data scraping, our client was able to identify consumer trends, preferential cuisines, and trends in eating out. It allowed them to fine-tune their product and service offerings in terms of menu selection to fit the needs of Hong Kong consumers.
Key Challenges
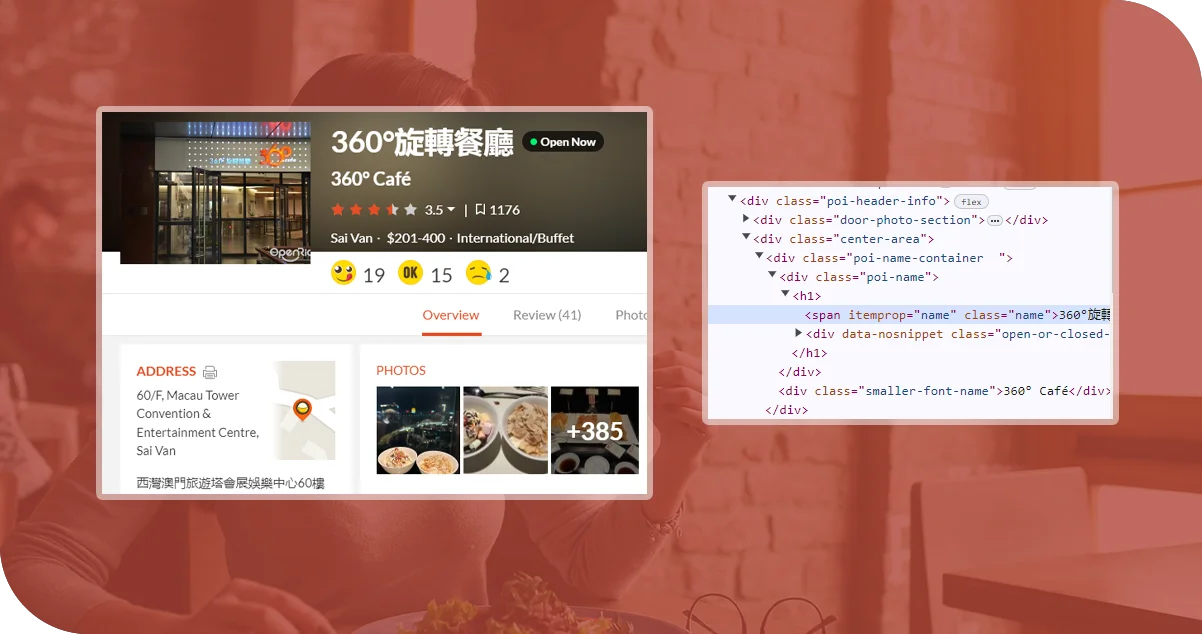
Data Privacy Concerns: Being a crowdsourcing site, OpenRice collects user-submitted content, which renders compliance with data protection acts and makes it challenging to preserve users' anonymity.
Unstructured Data: OpenRice information may need to be better organized; thus, unique methods, such as parsing, may be necessary to extract information efficiently.
Data Volume: Due to the massive amount of data on OpenRice, it becomes difficult to handle, sort, and analyze the collected data to extract valuable information for our client.
Key Solutions
Robust Data Extraction Algorithms: It is therefore clear that our restaurant data scraper has sophisticated routines to scrape for data from OpenRice since the data, primarily in unstructured form, presents some challenges to scraping.
Data Anonymization Techniques: To meet data privacy regulations regarding user-generated content presence in OpenRice, we use data anonymization approaches.
Scalable Infrastructure: Our OpenRice data scraper is designed with future capacity in mind, which is why we can handle large amounts of data from OpenRice and provide our clients with meaningful information about their business.
Methodologies Used
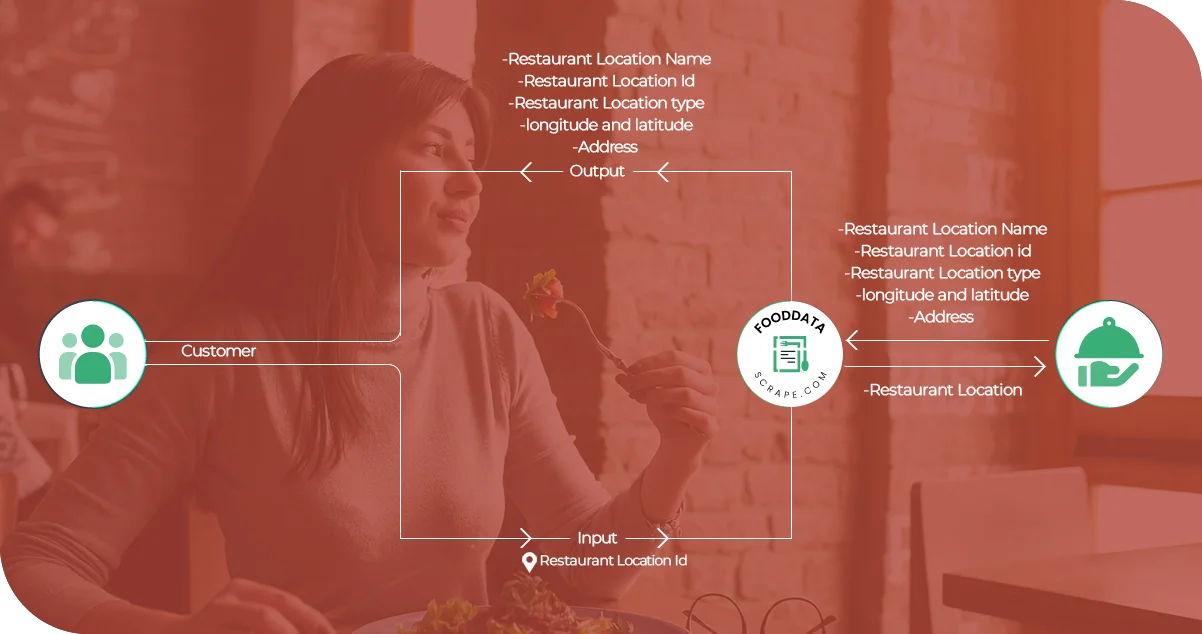
Natural Language Processing (NLP): Applying the NLP approach to extract specific information like restaurant name, location, cuisine type, and reviews from text data of OpenRice.
Image Recognition: Adding more value to the data by using image recognition technology to take data from images of menus, dishes, and restaurant interiors.
Sentiment Analysis: Using the sentiment analysis on the user reviews to determine the satisfaction levels and the sentiments of customers on certain restaurants or dishes.
Geo-location Tracking: Geo-location helps define dining preferences based on location to better understand specific geographic regions' eating habits.
Collaborative Filtering: Applying collaborative filtering to filter restaurants and serve the user's preferences and dining habits improves user engagement with the platform.
Advantages of Collecting Data Using Food Data Scrape
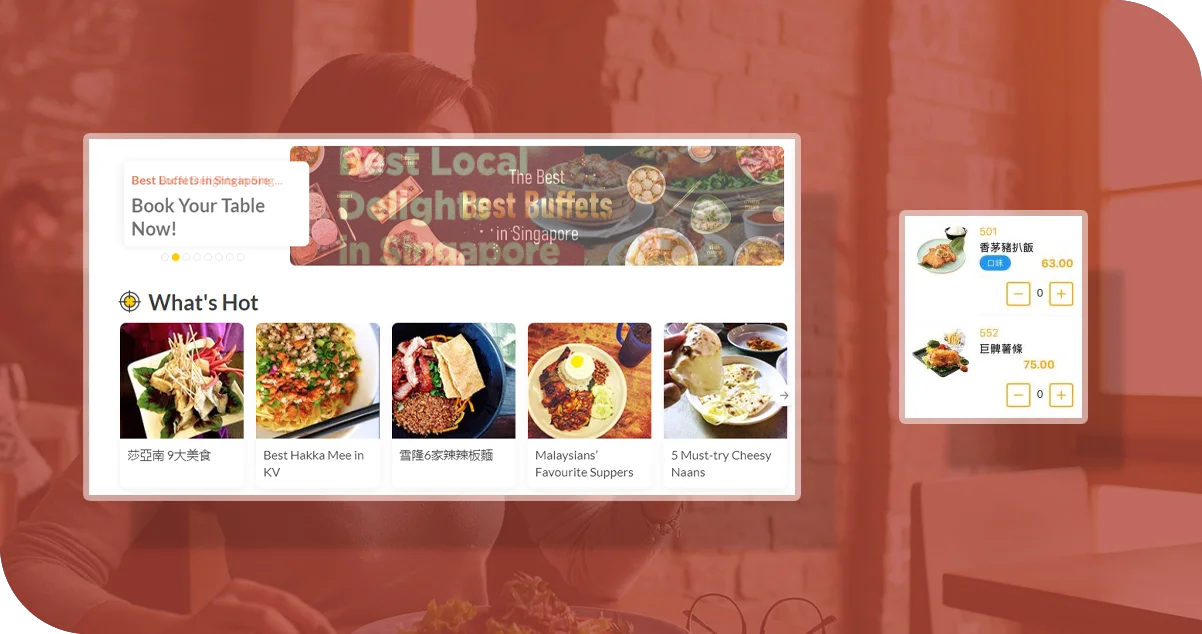
Comprehensive Insights: Food Data Scrape gives you access to many datasets, helping you learn as much as possible about the tendencies within the food industry and customers' preferences.
Real-time Updates: With this strategy, businesses can gather data, such as menu updates or promotions, on restaurant websites or food delivery platforms to help them maintain relevance with the market.
Competitive Analysis: It helps conduct a competitive analysis, where businesses can compare their food products, prices, and customers' feedback with their competitors to see which area they should focus on improving.
Increases Decision-making: By timely and properly attaining and analyzing data, organizations can make better decisions about their menu planning, pricing strategies, and marketing promotion, leading to better results and high returns.
Enhanced Customer Experience: Collecting information on customers' needs and expectations can help companies and organizations address their clients' needs more effectively, thus improving customer satisfaction and loyalty.
Final Outcomes: We were able to scrape OpenRice Hong Kong successfully, and as a result, we provided our clients with essential information about the local food industry and consumers to ensure that they make optimal decisions regarding adjustable strategies for increasing competitiveness.